Getting your Trinity Audio player ready...
|
By Janine Erasmus
Fifty years ago the concept might have been taken from a science fiction plot. Now it’s one of the new technologies that become more sophisticated as time passes. Using artificial intelligence (AI) and algorithms to track down suspicious activity is a reality – but can we trust them to reveal illicit financial flows?
“As systems and procedures continue to become more digitised, there are more opportunities to leverage available data to find the red flags that can indicate corruption and other integrity risks,” writes the World Bank’s Vinay Sharma in a blog post on AI as an anti-corruption tool. Sharma is the director for solutions and innovations in procurement. “But how can human beings sift through vast amounts of data to connect the dots necessary to pick up on every red flag that hints at corruption – without slowing down the government procurement process and ultimately public service delivery?”
And there are other questions. How exactly are the red flags produced? Do algorithms have bias? Can an algorithm ever truly replace a human?
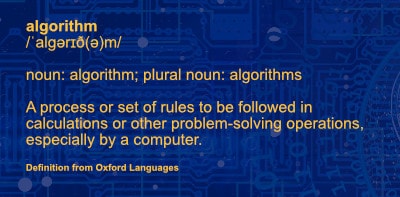
At the fourth Making Transparency Possible conference on 28 October 2020, data scientist Lars Erik Bolstad and AI research expert Anis Yazidi spoke on this topic. The conference took place under the theme Follow the money, and was held virtually at the Oslo Metropolitan University in Norway.
Bolstad works in the anti-money laundering (AML) unit of Den norske Bank – the same bank through which millions of Namibian dollars left that country in the Fishrot scandal. He is currently developing machine learning to detect suspicious behaviour in transaction data.
Asked how money laundering can be detected using machine learning, he explains that machine learning is a relatively new development in the field. “The traditional way for transaction monitoring is by using a rule engine,” he says. “Essentially there are a number of rules, and an engine matches transactions to those rules and fires off alerts when appropriate.”
One clear challenge for this technology, he adds, is that it is not accurate enough. “It generates a large number of false positives or false alerts which have to be manually investigated, and that is very demanding on human resources.”
Another challenge is that such a system will typically only be able to detect fairly trivial patterns or behaviours, says Bolstad. “So this is where machine learning comes in and the use of more advanced methodologies. One potential use of this technology is to try to automate a portion of the very resource-demanding manual work, but the other obvious angle is to detect more complex patterns of behaviour than what the rule engine is able to.”
Sharma, also aware that manual analysis of massive datasets is impractical and a drain on human resources, notes the potential of AI to digest huge and diverse datasets, and detect patterns that hint at the possibility of corrupt behaviour in relation to, say, public spending. This, he says, would allow analysts to “better map networks of relations, locations, use of shell companies, off-shore jurisdictions, and banking information of bidders to address potential risks before a contract is issued. These are just a few examples of indicators that can be assessed to reveal potential concerns”.
Furthermore, he argues, if the data that the World Bank holds on procurement can be combined with datasets from other international organisations, national procurement data, and beneficial ownership or other corporate information, “we can gain greater insight on how to make better decisions on public spending to assure greater value for money and mitigate the corrosive effects of corruption”.
What’s even more exciting, Sharma says, is the potential to take this exercise to scale and make such a tool available to governments worldwide.
As exciting as it is, however, the notion still holds great challenges.
Bias can cause inaccurate results
Prof Anis Yazidi leads the applied AI research group at the Oslo Metropolitan University. “The role of artificial intelligence is to automate the cognitive process of humans, to imitate the human capabilities of reasoning,” he explains.
“The advantage of AI is processing a lot of information that is overwhelming for humans – we can’t manually go through millions of transactions, but the machine can.”
He distinguishes between two types of machine learning algorithms – supervised learning and unsupervised learning. “With supervised learning, you have data that is labelled with the ground rules. This means that you can identify some bank transactions as money laundering and other transactions as acceptable, according to the rules.”
Unsupervised learning is where the data is provided but there are no rules to indicate which transactions are legitimate and which are not. In this case anomalies or deviations from the data produce the red flags.
The problem with supervised learning, Yazidi says, is that bias may creep in because humans program the system. “Let’s say we have a system based on AI to decide whether I get a loan or not. In this system we have very good predictive ability if I take my social status, for example, into consideration, because we know that people coming from poor areas or regions might have problems to pay. But if loans are given on this basis without looking at the person themselves, favouring some people and not others, this goes against the principle of people having equal chances.”
So the system might be good at making its predictions, he says, but at the same time it’s unfair because it produces more flags for some categories of people. It should apply the same principles across all categories.
“We might be oversampling from some subcategories, for example, companies or people from a certain region, and we might therefore be finding more unlawful tendencies. But what we are doing is putting bias into the system.”
When using unsupervised learning, where the system is not told which data is legitimate and which is not legitimate, the risk of bias is significantly decreased.
Bolstad agrees that bias is a fundamental challenge in working with data and algorithms and machine learning. “The models we use for AML differ from those use for applying for a loan. We use machine learning models for risk classification on customers but the prediction or classification from this model will always be subject to a manual process afterwards, so there’s no automatic consequence or impact for the customer. We’re nowhere near automatically reporting a customer based on the machine learning algorithm.”
The current expectations for artificial intelligence are a little ambitious, adds Yazidi. “I might be proven wrong in 5 or 10 years but AI will not surpass our human capacity. It will just automate some tasks that we human beings are doing. AI does the same cognitive process that we do, but much faster and using fewer computational resources.”
Human beings are undoubtedly able to make the same connections that an AI system can, given enough time – but time is a luxury in AML and the policing of other crimes, since criminals may escape with their ill-gotten gains if not swiftly apprehended.
Significant challenges to overcome
The exact path an algorithm takes to arrive at its conclusion is not always clear, making it more challenging to fine-tune the journey. “We get the conclusion but the explanation is a problem,” says Yazidi.
Sometimes the decision might be based on a factor that is not related to the question, he adds, citing the example – considered an urban legend because there is no verifiable source for it – of a neural network in the Cold War that had learned to detect camouflaged tanks in photographs by analysing the photos to detect even small parts of the tank that were visible. The task succeeded, but researchers later realised the training photos of camouflaged tanks had been taken on cloudy days, and photos with no tanks had been taken in sunny weather. The neural network had therefore learned the difference between the cloudy and sunny backgrounds, instead of actually spotting camouflaged tanks.
Fortunately this is all a learning process, Yazidi says, and there many things that can be improved to give more credibility to conclusions reached. One of these measures is to ensure that the dataset used is representative enough of all the different categories under scrutiny so there is no imbalance. Another is to be careful of programmers polluting the data because of perceptions and prejudices, thereby giving the wrong examples for the system to reflect in its conclusions.
Banks could use AI more effectively to stop money laundering
Bolstad dismisses the notion that banks deserve the criticism they get in this respect. “There’s a lot of work going on to become better at detecting suspicious financial behaviour, to model data in different ways, to improve existing systems and introduce more advanced methodologies. The intention is to be as good as possible.”
Others feel differently. Ben Charoenwong, assistant professor of finance at the National University of Singapore, notes that banks have more than enough data to detect anomalies that stand out amidst a series of transactions. But banks are known for facilitating money laundering, and for managing the dirty money of shady characters linked to terrorism and crime, and therefore their AML and Know Your Customer (KYC) efforts will not succeed.
“Effectively, the big banks failed in using that existing rich dataset to improve the AML/KYC process. There is only one reason for this failure: the AML/KYC process is not a matter of data science. It is a matter of incentives … banks make too much money violating these processes.”
He cites the example of HSBC’s brazen money laundering activities for Saudi and Bangladeshi clients tied to terrorist activities – but though the US and UK governments blacklisted these clients, the bank simply bypassed the alert system by replacing spaces in the clients’ names with dots.
“Surely any computer scientist worth a dime would have included condition checks on the string, normalising them so that the strings are comparable and robust to small permutations of names,” Charoenwong says. “Even without a hard match, the algorithm should produce more useful flags … but despite that, ‘no one’ picked up on this (or some people did and were subsequently silenced). It seems…almost intentional.”
Charoenwong is equally unconvinced by lawyers and administrative staff who, charging by the hour, “do not have an incentive to make the process quicker. In the sea of ever-growing expanding and increasing regulatory complexity, the lawyers are the mainstay winners. It is based on the willpower of those in charge of conducting compliance, and big banks will not exhibit that willpower.”
His sentiments are echoed – though not quite as cynically – by Nils Köbis of the University of Amsterdam, and Christopher Starke of the Heinrich Heine University in Düsseldorf. The two post-doctoral researchers presented a paper at the European Consortium for Political Research’s (ECPR) 2020 general conference, on Artificial Intelligence as an Anti-Corruption Tool – A Conceptual Framework. Their abstract notes the use of recent AI developments to find innovative solutions for existing problems – for example, AI-based systems have been used to diagnose specific forms of cancer more efficiently.
“Against this backdrop, anti-corruption agencies are lagging in their quest to tap into the potential of AI to fight corruption,” they state, and despite case studies on this specific use of AI, there is no coherent conceptual framework.
The framework advanced by Köbis and Starcke demonstrates the potentials and perils of existing applications, and identifies existing knowledge gaps in the academic and policy literature at the nexus of AI and anti-corruption.
Future AI technologies, they say, hold immense potential for anti-corruption – not least because they can overcome the problem of agencies themselves falling prey to corruption. But at the same time, “these technologies pose new ethical challenges, such as increased risks of privacy breaches and surveillance”.
To responsibly harness the power of AI, they advise, society must be in the loop and incorporating citizens’ voices is key – one example is the crowdsourcing of public opinion on the use of autonomous vehicles. Using similar approaches can help to involve citizens in the development and adoption of AI in anti-corruption.
“Since AI [for anti-corruption] is still in its initial phase such a citizen-centred approach can help to shape its future trajectory, so that AI tools can fulfil some of the hopeful aspirations in the fight against corruption.”